How to Use AI for Data Processing without Violating the GDPR: CNIL Guidelines and Action Plan
Discover CNIL's guidelines on deploying AI while ensuring GDPR compliance. Learn key principles, legal bases, data handling, and the action plan shaping AI's future. Dive in now!
CNIL, the French data protection authority, has published guidelines on the use of artificial intelligence along with an action plan.
The guidelines consist of several resources for data controllers and processors who involve AI in personal data processing. The resources provide directions on how to use AI for personal data processing without violating the GDPR.
The action plan, on the other hand, is focused on the work CNIL plans to do in the following period. As a supervisory authority, the CNIL has the duty to oversee the use of AI and direct businesses toward compliant use of the technology.
In this article, we will delve into what is required from data controllers and processors regarding the use of AI where personal data processing is involved. It will give you an idea of how to approach AI processing with minimal risks.
In the end, we'll get into the CNIL action plan to get an idea of regulators' plans for the future and where they are headed.
How to use Artificial Intelligence without violating the GDPR?
To ensure GDPR compliance in building or deployment of AI systems that respect the privacy of individuals, you need to take care of the following:
Defining the purpose of the AI system
For AI systems using personal data to align with the GDPR, they must be designed, trained, and used with a specific goal in mind. This goal should be set during the early design phase, align with the organization's objectives, and be easily understood.
AI systems based on machine learning undergo two main phases:
- Learning Phase, which includes creating and training the AI system, resulting in a model that represents the system's learnings from the training data.
- Production Phase, which is the actual use of the AI system developed in the first phase.
From a data protection perspective, these phases have different objectives and should be distinct. Therefore, you as an AI model creator can have different objectives in each stage. For both phases, however, the reason for processing personal data should be specific, valid, and transparent.
Establishing a legal base for the data processing
The General Data Protection Regulation (GDPR) of the EU does not allow the processing of personal data without a legal basis. As a result, you need to have one for any AI system processing personal data.
The GDPR provides six legal grounds: consent, legal obligation compliance, contract performance, public interest, vital interests, and legitimate interest.
If the training of an AI system involves the processing of personally identifiable data, you need to have a legal basis. In many cases that would be explicit user's consent.
While innovative AI systems fundamentally follow the same rules as other personal data processing, they have their own specifics that require additional attention. Specifically, AI systems, especially those using machine learning, first use data in a learning phase and then in an operational phase. AI systems must not use unlawfully gathered personal data in either phase.
Compiling a database of training data
AI systems, especially machine learning-based ones, need large amounts of data for training, assessment, benchmarking, and validation. The creation of datasets for AI training involves collecting new personal data specifically for this or re-using data initially gathered for another reason.
When collecting new personal data for training AI, it is crucial to:
- Have a legal basis for doing so, which in most cases would mean obtaining explicit consent, and
- Inform users what you are going to do with their data.
For re-using existing data in artificial intelligence system training, you have to ensure that the training itself is in line with the purposes the data has been collected in the first place. If it is not, then you need to obtain consent from the data subject to use it. For example, the CNIL has approved re-using video surveillance footage for a study on crowd movement patterns, a computer vision task, considering it in line with the original purpose. However, it required the data controller to inform the individuals whose data had been processed.
Regarding transparency, CNIL recommends organizations to inform users on data use for AI training before collecting the data, or within a month after receiving datasets from third parties.
Data minimization
GDPR is clear that only the minimum amount of data shall be processed for the intended purposes. This is called the data minimization principle.
Although modern AI systems, especially powerful machine learning ones, thrive on vast data volumes, data minimization doesn't hinder such processing.
Before processing the data, you need to determine what data categories are absolutely essential for training the AI. Then, you can process only that data for such purpose. It must respect the proportionality principle.
Different phases may need different amounts of data, The learning phase, which aims to explore machine learning capabilities, might need vast data, some of which might be redundant during deployment.
The CNIL further provides the example of a pharmaceutical company that wanted to train the AI model to find variables explaining prostate cancer. They denied the pharmaceutical lab's request to process data of the entire active patient population from various centers' medical records, including those not having prostate cancer and even women's records. Processing this data, justified by the need for "true negatives" for training a classifier, seemed disproportionate and unnecessary for creating an effective AI system. Therefore, the data minimization principle could not be met and the processing would not have been proportional.
Data retention
You cannot store personal data forever, but only as long as you need it for specific processing purposes, in this case, the training and deployment of an AI model.
The GDPR requires AI operations to define a data retention period aligned with the purpose. For instance, if the goal is performance measurement, it should be well-planned, and only relevant data should be kept longer. Merely wanting to measure performance over an extended period doesn't justify retaining all data for long durations.
However, for AI systems the CNIL is flexible in allowing extended data retention periods because there might be a need to retain personal data longer than other processes. This could be for creating datasets for training, developing new systems, or ensuring traceability and gauging performance over time during production.
If the data is stored for scientific research purposes, it can be kept for extended periods.
Supervision and continuous improvement
The line between learning and production phases in AI isn't always evident, especially in continuous learning systems. In these systems, data from the production phase also refines the system, forming a feedback loop. The relearning can occur at various intervals, such as hours, days, or months, based on the goal.
Continuous learning is essential in the development of AI, but it poses risks like introducing bias or performance decline. The CNIL suggests that using data for dual purposes (system improvement and its primary function) brings up data protection concerns:
- Can the two purposes be distinctly separated?
- Is it feasible to differentiate the learning and production phases always?
- If a third-party data controller uses an algorithm from a provider, how should responsibilities for both processing phases be divided?
In its decisions, the CNIL has consistently believed that the learning and production phases can be separated, even if closely linked. In the example of voice assistants, the CNIL discussed reusing data from voice assistants to enhance the service. They highlighted the annotation of new learning examples to boost AI performance, distinguishing this from the service provided to the voice assistant user, therefore separating the processing purposes.
Regarding responsibility division between AI system providers and data controllers, the CNIL has addressed the reuse of data by a data processor given by a data controller. In general, the reuse of data is allowed for AI systems. A system provider can legally reuse data if they meet several conditions:
- Getting permission from the data controller to reuse the data
- Compatibility testing
- Being transparent and informing individuals
- Respecting data subject rights, and
- Ensuring the compliance of the new processing activity.
Safeguards for the AI model risks
Like any other online property, AI models are under threat of an attack. Common threats include membership inference attacks, model evasion attacks, and model inversion.
For instance, many studies found that large language models can memorize specific textual elements they're trained on, such as names or credit card numbers. And that's where the risks lay. These risks require further technical and organizational measures for mitigation of the risks.
If, in a worst-case scenario, an AI model faces a successful privacy attack and personal data is exposed, that would be a data breach. CNIL suggests that such a model must be promptly removed.
Providing information to users
The GDPR's transparency principle requires businesses to inform users on the processing of their data. That information must be concise, clear, understandable, and easily accessible, using straightforward language, as the GDPR requires.
Data subjects must be informed of the processing at the moment of the collection of data. But, having in mind that some AI models are trained on data gathered from all the corners of the internet, it is challenging to inform all of the data subjects.
CNIL, however, says that in situations where data isn't directly collected from individuals, the right to be informed might be exempted, especially if informing them is impossible or demands excessive efforts, like in AI processing for scientific research.
Exercising of data subject GDPR rights
Individuals have data subject rights. The data controller must explain them on how to exercise these rights, and individuals should receive a response within a month.
AI systems that process personal data, must meet the GDPR principles for individuals' rights. These rights protect individuals from the consequences of an automated system, allowing them to understand and if needed, challenge related data processing. These rights are applicable throughout the AI system's lifecycle - both for the learning and during the production phase.
During the AI design phase, data controllers shall plan for potential data protection issues, such as responding to data subject requests.
AI models can also contain personal data:
1. Intentionally, as seen in specific algorithms containing parts of training data. If the data controller can re-identify the individual, their rights should be exercised.
2. Accidentally, as mentioned in the risks associated with AI models. In this case, exercising the rights might be impossible and the CNIL allows for declining the request and explaining to the user that exercising the right is impossible.
Supervising automated decisions
Aside from other rights of individuals, individuals have the right to avoid fully automated decisions, often stemming from profiling, that have significant legal or personal impacts. Organizations can only automate such decisions if:
- The user provides explicit consent;
- The decision is contractually necessary; and
- The decision is legally authorized.
Whatever the legal basis, users have the right to:
- Know about the automated decision;
- Understand the decision-making logic;
- Challenge the decision and share their perspective; and
- Request human intervention for decision review.
This is why data controllers must plan for human intervention, allowing individuals to review their situation, understand the decision, and contest it.
Assessing the system
Assessing AI systems is important, especially in the context of the EU's AI Act - draft regulation. From a perspective of compliance with the GDPR, the assessment ensures that the AI ecosystem works as intended and doesn't harm individuals.
In the guidelines, CNIL publishes an example of an experiment involving facial recognition technology, the CNIL required a detailed assessment protocol to measure the technology's exact contribution. To give you an idea of what they required to know, here's a list:
- Standard performance metrics recognized by the scientific community;
- A thorough analysis of system errors and their operational consequences;
- Details about the experiment's conditions (e.g., lighting, weather, image quality, obstructions);
- Information on potential discrimination risks with the AI system's deployment; and
- Insights into the system's implications in an operational setting, considering real-world scenarios (e.g., the impact of a 10% false positive rate for 10 alerts versus 1,000 alerts).
Avoiding algorithmic discrimination
Garbage in, garbage out. You need to ensure that you don't include discriminatory data in the AI model and that the algorithms do not discriminate.
There are two types of issues here:
1. Learning Data Issues. Data might be non-representative or, as CNIL says - even if it mirrors the "real world", it might inherently be discriminatory (like perpetuating gender pay disparities).
2. Algorithmic Flaws. The algorithm could lead to biased outcomes by design.
CNIL provides an example to better understand algorithmic discrimination: during an evaluation of an organization using an automated system to assess video CVs, the CNIL identified a discriminatory bias because the AI failed to account for the variety of candidates' accents. The CNIL does not explain what exactly this failure means and how the system should have behaved.
What are the CNIL AI Action Plan objectives?
The CNIL also published an action plan
- Understanding AI's Impact. The CNIL will study how AI systems work and their effects on people. They'll look into protecting public web data from scraping, safeguarding data shared by AI system users, and understanding the implications for people's rights concerning data used to train AI and the results AI systems give.
- Guiding AI that Protects Personal Data. The CNIL aims to help organizations create AI that respects personal data. They'll release advice and best practices on AI topics, like rules for sharing and re-using data and designing generative AI systems.
- Supporting AI Innovators in France and Europe. The CNIL wants to help those in the AI field innovate while protecting French and European rights. They'll start a new project call for their 2023 regulatory sandbox and talk more with research groups, R&D centers, and AI system developers.
- Checking and Overseeing AI Systems. The CNIL is working on a tool to check AI systems. They'll also keep looking into complaints about AI, including generative AI.
Final thoughts: creating and using privacy-friendly AI and ensuring GDPR compliance
If you are involved in the development of artificial intelligence systems, you have no easy task in regard to the GDPR and the French Data Protection Act. All we have for now are general recommendations, no case law, and no clear way ahead. Designing a privacy-friendly AI system is not difficult, but if your systems require the processing of personal data, you have to take into account the application of the GDPR into your system.
A good starting point is conducting a Data Protection Impact Assessment, where you'll lay down all the risks and determine mitigation strategies. We have a comprehensive article on that topic that could help. Combine that with the CNIL Self-assessment guide for artificial intelligence (AI) systems and you'll be very close to meeting the GDPR requirements for AI.
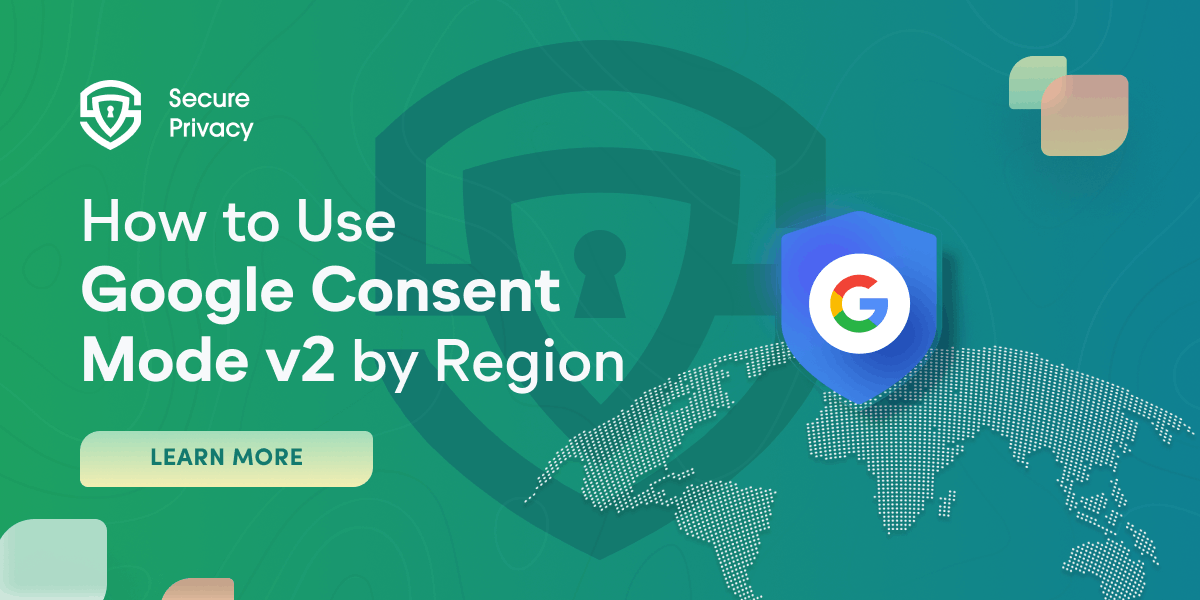
How to Use Google Consent Mode v2 Outside the EEA and the UK
Discover why using Google Consent Mode v2 outside the EEA and the UK might be unnecessary. Learn about compliance requirements, the impact on data collection, and how to optimize your approach based on regional privacy laws.
- Europe GDPR
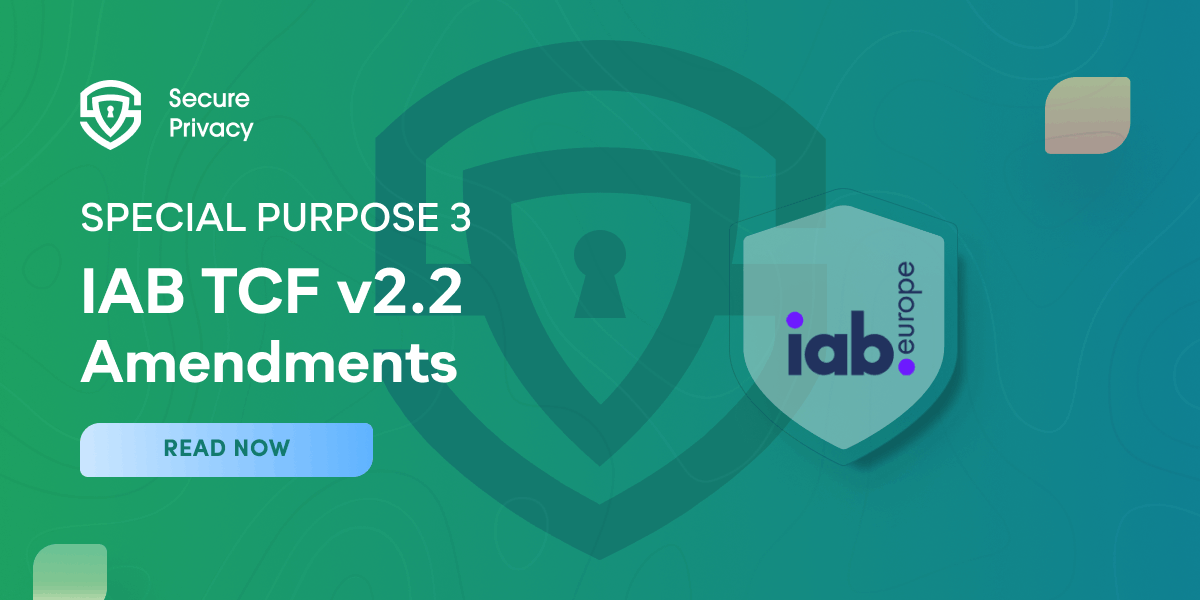
The Impact of Special Purpose 3: Latest Amendments to the IAB Transparency and Consent Framework (TCF) V2.2 Policies by IAB Europe
Discover how the latest amendments to the IAB Transparency and Consent Framework (TCF) V2.2, particularly the introduction of Special Purpose 3 (SP3), are transforming user consent and transparency in the digital advertising ecosystem. Learn about the new requirements for protecting children's privacy, preventing dark patterns, and ensuring explicit consumer consent.
- Europe GDPR
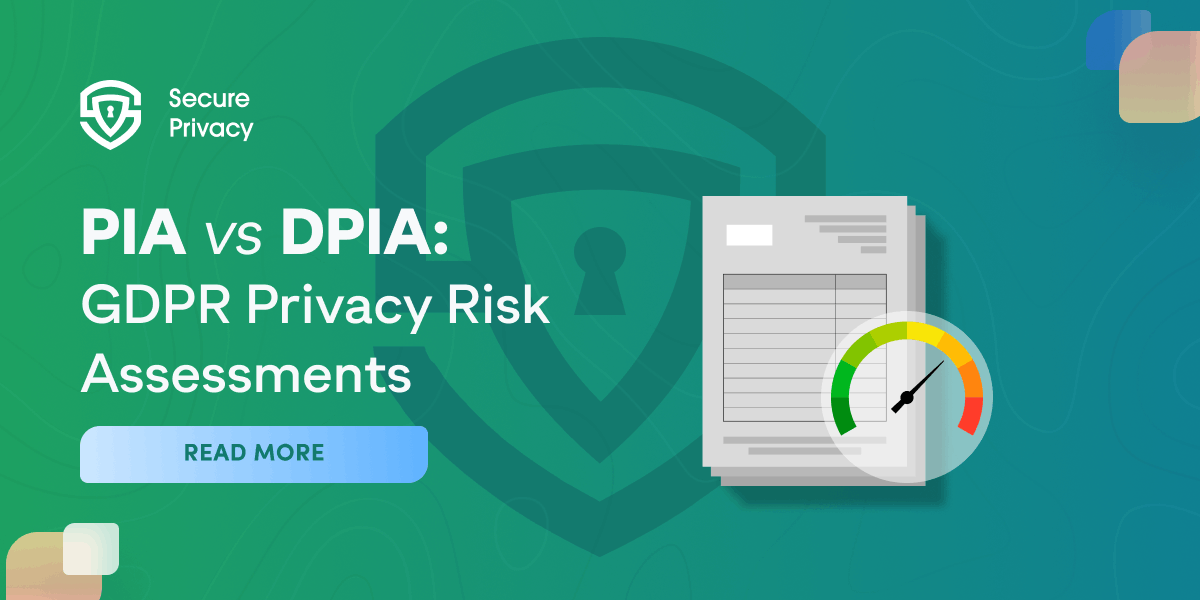
Understanding the Difference Between a PIA and DPIA in GDPR Privacy Risk Assessments
Learn the key differences between Data Protection Impact Assessments (DPIAs) and Privacy Impact Assessments (PIAs). Understand their importance in ensuring compliance with privacy laws and best practices for mitigating privacy risks.
- Europe GDPR
- Data Protection