When AI Makes Decisions: Your Right to Know Why
You apply for a mortgage and get rejected. An algorithm flags your insurance claim as potentially fraudulent. A hiring system screens out your resume before human eyes ever see it. In each case, artificial intelligence made a decision affecting your life—but can you find out why?
The "right to explanation" addresses this question directly, establishing your entitlement to understand decisions made by AI systems. As algorithms increasingly determine who gets loans, jobs, healthcare, and other vital services, this right becomes essential for protecting individuals from automated discrimination and errors.
European regulations like GDPR and the EU AI Act have established the most comprehensive frameworks for this right, but implementing meaningful explanations faces significant challenges. Here's what you need to know about demanding explanations when AI makes decisions about you.
Why Explanations Matter for AI-Generated Content
The right to explanation isn't just about fairness—it addresses fundamental power imbalances between individuals and AI systems.
From Black Boxes to Transparent Decisions
Most advanced AI systems operate as "black boxes" where even their creators can't fully explain specific decisions. This opacity creates several problems:
- Undetected bias: Without explanations, discriminatory patterns remain hidden
- Inability to appeal: You can't effectively challenge decisions you don't understand
- Limited recourse: Systems can't improve without identifying specific failure points
- Diminished agency: People lose control over decisions affecting their lives
When AI generates content like credit assessments, tenant screening reports, or hiring recommendations, explanations provide essential accountability and the opportunity to correct errors.
Real-World Impact of Unexplainable Decisions
Consider these scenarios:
- A tenant rejected by an AI screening system can't address the specific concerns that led to the rejection
- A patient denied insurance coverage for a medical procedure doesn't know which factors triggered the denial
- A loan applicant faces higher interest rates without understanding which aspects of their financial history affected the algorithm's decision
Without explanations, individuals face what legal scholars call "algorithmic alienation"—being subject to consequential decisions they cannot understand or effectively challenge.
Legal Frameworks Establishing the Right to Explanation
Several major regulations have established various forms of the right to explanation, though with significant differences in scope and enforceability.
GDPR Article 22: The Foundation
The European Union's General Data Protection Regulation provides the most established legal basis for explanation rights, particularly in Article 22, which addresses "automated individual decision-making."
Key provisions include:
- General prohibition: Decision-making based solely on automated processing is prohibited when it produces legal effects or similarly significant impacts
- Limited exceptions: Automated decisions are permitted only when necessary for contracts, authorized by law, or based on explicit consent
- Mandatory safeguards: Even when permitted, controllers must implement measures allowing individuals to "obtain human intervention, express their point of view, and contest the decision"
- Information requirements: Controllers must provide "meaningful information about the logic involved" in automated decisions
These provisions directly support the right to explanation by ensuring individuals can meaningfully challenge automated decisions affecting them.
The EU AI Act: Risk-Based Transparency
The EU AI Act builds on GDPR's foundation with more specific transparency requirements tailored to different AI applications.
The Act creates a tiered approach to transparency based on risk levels:
- High-risk AI systems: Face the most stringent explanation requirements
- General-purpose AI models: Require special transparency guardrails given their versatility
- Specific AI applications: Additional disclosure requirements for certain systems regardless of risk level
For AI-generated content specifically, the Act establishes several important transparency rules:
- AI interaction disclosure: Systems must clearly indicate when individuals are engaging with AI rather than humans (unless already obvious)
- Emotion recognition notification: People must be informed when subjected to emotion recognition or biometric categorization
- Deepfake labeling: Content mimicking reality through AI-generation or manipulation must be disclosed as artificial
These requirements directly address concerns about deceptive AI-generated content by ensuring people know when they're viewing algorithmically created material.
The Gap Between Legal Rights and Practical Reality
Despite these regulations, meaningful explanations remain elusive for many AI systems. Several challenges complicate implementation:
Technical Limitations
The most accurate AI systems often use complex approaches like deep neural networks, which process information in ways that resist straightforward explanation. These systems may have:
- Millions of parameters interacting in non-intuitive ways
- Feature interactions that create emergent behaviors
- Statistical correlations without clear causal relationships
These characteristics make producing useful, human-understandable explanations technically challenging, particularly for general-purpose AI systems.
Business Resistance
Companies deploying AI systems often resist comprehensive explanations due to:
- Trade secrets: Detailed explanations might reveal proprietary algorithms or training methodologies
- Gaming concerns: Full transparency could enable people to manipulate systems
- Performance tradeoffs: More explainable models sometimes sacrifice accuracy
These business interests create tension with individuals' rights to understand decisions affecting them, particularly when explanations might reveal problematic patterns.
Enforcement Gaps
Even where right to explanation exists legally, practical enforcement faces obstacles:
- Individuals often don't know when AI made a decision about them
- Technical complexity makes evaluating explanation adequacy difficult
- Regulatory agencies have limited technical expertise and resources
- Global deployment of AI systems creates jurisdictional challenges
These gaps mean that even strong legal protections may provide limited practical benefit without corresponding enforcement mechanisms.
What Meaningful Explanations Should Include
Not all explanations are equally valuable. A truly meaningful explanation should provide:
Counterfactual Understanding
Effective explanations answer the question: "What would I need to change to get a different outcome?" For example:
- "Your loan application was denied primarily because your debt-to-income ratio exceeds our threshold of 43%. Reducing your monthly debt obligations by $300 would likely change this decision."
- "Your resume was screened out because you lack the required 3 years of experience with Java programming specified in the job requirements."
This counterfactual approach helps individuals understand both the reason for the decision and what actions might produce different results.
Contextual Relevance
Explanations should match the recipient's needs and technical understanding:
- A consumer needs different information than a regulator or technical auditor
- Technical jargon should be replaced with accessible language
- Visual representations often communicate complex patterns more effectively than text
The best explanation systems adapt their communication to the specific context and audience.
Actionable Insights
Truly useful explanations enable concrete actions, such as:
- Correcting inaccurate input data
- Understanding which factors can be modified
- Identifying potential system mistakes or biases
- Providing grounds for meaningful appeals
Without these actionable elements, explanations become merely technical exercises rather than practical tools for recourse.
Implementation Approaches for Different Sectors
Different industries face unique challenges in implementing the right to explanation.
Financial Services: Building on Existing Frameworks
Financial services have a head start on explanation requirements through regulations like the Equal Credit Opportunity Act, which already mandates specific reasons for credit denials. Effective approaches include:
- Expanding existing adverse action notices to include algorithmic factors
- Developing interactive tools that allow consumers to explore how different financial choices affect their outcomes
- Creating standardized explanation formats for common financial decisions
These approaches leverage existing compliance frameworks while addressing the unique challenges of AI-driven decisions.
Healthcare: Balancing Effectiveness and Understanding
For healthcare applications, explanations must balance technical accuracy with patient comprehension:
- Clinical decision support systems should clarify which patient-specific factors influenced recommendations
- Insurance coverage determinations need clear explanations of policy rules applied by algorithms
- Diagnostic AI should indicate confidence levels and alternative considerations
These explanations require close collaboration between medical and technical experts to ensure both accuracy and accessibility.
Hiring and Employment: Addressing Power Imbalances
Employment contexts present particular challenges due to information asymmetry between employers and candidates:
- Automated resume screening systems should provide specific qualification gaps
- Performance evaluation algorithms need transparent criteria and weighting
- Promotion recommendation systems must clarify which achievements factored into decisions
These applications require special attention to potential discrimination and the profound impact of employment decisions on individuals' lives.
Emerging Best Practices for Developers
Organizations building explanation capabilities into AI systems should consider these approaches:
Design for Explainability From the Start
Rather than treating explanations as an afterthought, incorporate them into the design process:
- Select model architectures that balance performance with interpretability
- Document design choices, training data, and key parameters
- Create explanation interfaces alongside core functionality
- Test explanations with actual users before deployment
This "explanation by design" approach avoids the difficulties of retrofitting explanations onto black-box systems.
Implement Layered Explanation Systems
Not all explanations serve the same purpose. Effective systems provide multiple layers:
- First layer: Simple, accessible explanations for affected individuals
- Second layer: More detailed information for those who request it
- Third layer: Comprehensive technical documentation for auditors and regulators
This approach satisfies both casual inquiries and rigorous examination while respecting different levels of technical understanding.
Conduct Regular Explanation Audits
Explanations themselves require ongoing evaluation:
- Test whether explanations accurately reflect system behavior
- Verify that non-technical users can understand and act on explanations
- Ensure explanations remain valid as systems and data evolve
- Check for potential disclosure of sensitive information
These audits help maintain the integrity of explanation systems over time and across system updates.
The Future of AI Transparency
As AI systems become more sophisticated and widespread, explanation requirements will continue evolving.
From Individual to Collective Explanations
The future likely involves a shift from purely individual explanations to broader systematic transparency:
- Algorithmic impact assessments describing overall system behavior
- Representative explanations showing typical decision patterns
- Aggregate statistics revealing potential demographic disparities
- Public registries of high-risk AI systems with standardized documentation
These collective approaches complement individual explanations by exposing patterns that might not be visible in single cases.
Technical Innovations in Explainability
Researchers are developing new approaches specifically designed for explanation:
- Local interpretation methods that explain individual decisions
- Global interpretation techniques revealing overall system behavior
- Interactive explanation interfaces allowing users to explore different scenarios
- Natural language explanations that translate technical details into accessible terms
These innovations may eventually bridge the gap between complex AI systems and meaningful human understanding.
Conclusion: Toward Accountable AI
The right to explanation represents a critical safeguard against potential harms of automated decision-making. While technical and business challenges complicate implementation, providing meaningful explanations is essential for maintaining human autonomy in an increasingly algorithmic world.
As AI-generated content and automated decisions become more prevalent, the importance of explanation rights will only grow. Effective explanations enable individuals to understand, challenge, and potentially correct decisions affecting their lives—ensuring that AI systems remain tools for human benefit rather than opaque arbiters of opportunity.
The path forward requires balancing innovation with accountability, efficiency with fairness, and technological advancement with human dignity. By developing robust explanation frameworks now, we can shape AI systems that make consequential decisions transparently, allowing affected individuals to understand not just what was decided, but why.
Frequently Asked Questions
Do I have a legal right to explanation for all AI decisions about me?
Not universally. In the European Union, GDPR provides limited explanation rights for automated decisions with significant effects, while the AI Act establishes additional transparency requirements for specific AI systems. In the United States, explanation rights exist in certain domains (like credit decisions under the Equal Credit Opportunity Act) but not comprehensively. Many countries have no established explanation rights for AI decisions.
What's the difference between transparency and explainability?
Transparency typically refers to general information about how an AI system works, including its purpose, capabilities, and limitations. Explainability focuses on specific decisions, providing reasons why the system reached a particular conclusion in an individual case. While related, they serve different purposes—transparency enables general oversight, while explainability allows individuals to understand decisions affecting them personally.
Can companies refuse to explain AI decisions by claiming trade secrets?
Companies often cite intellectual property concerns when limiting explanations, but this argument holds varying weight depending on jurisdiction and context. Under GDPR, trade secrets cannot completely override explanation rights, though they may limit the detail provided. Regulators increasingly reject blanket trade secret claims, requiring companies to balance proprietary interests with individual rights.
What should I do if I'm denied an explanation for an AI decision?
Start by formally requesting an explanation from the organization that made the decision, specifically referencing any applicable regulations (like GDPR Article 22 in Europe). If unsuccessful, contact your national data protection authority or consumer protection agency. Document all communications carefully. For specific sectors like financial services or healthcare, industry-specific regulators may provide additional assistance.
Are some AI systems too complex to explain?
While some advanced AI systems like deep neural networks present significant explanation challenges, researchers have developed various techniques to provide meaningful information about their decisions. The technical difficulty of explanation should not be used as a blanket exemption from accountability. Even complex systems can offer useful insights about the primary factors influencing their decisions, even if complete explanations remain elusive.
Get Started For Free with the
#1 Cookie Consent Platform.
No credit card required
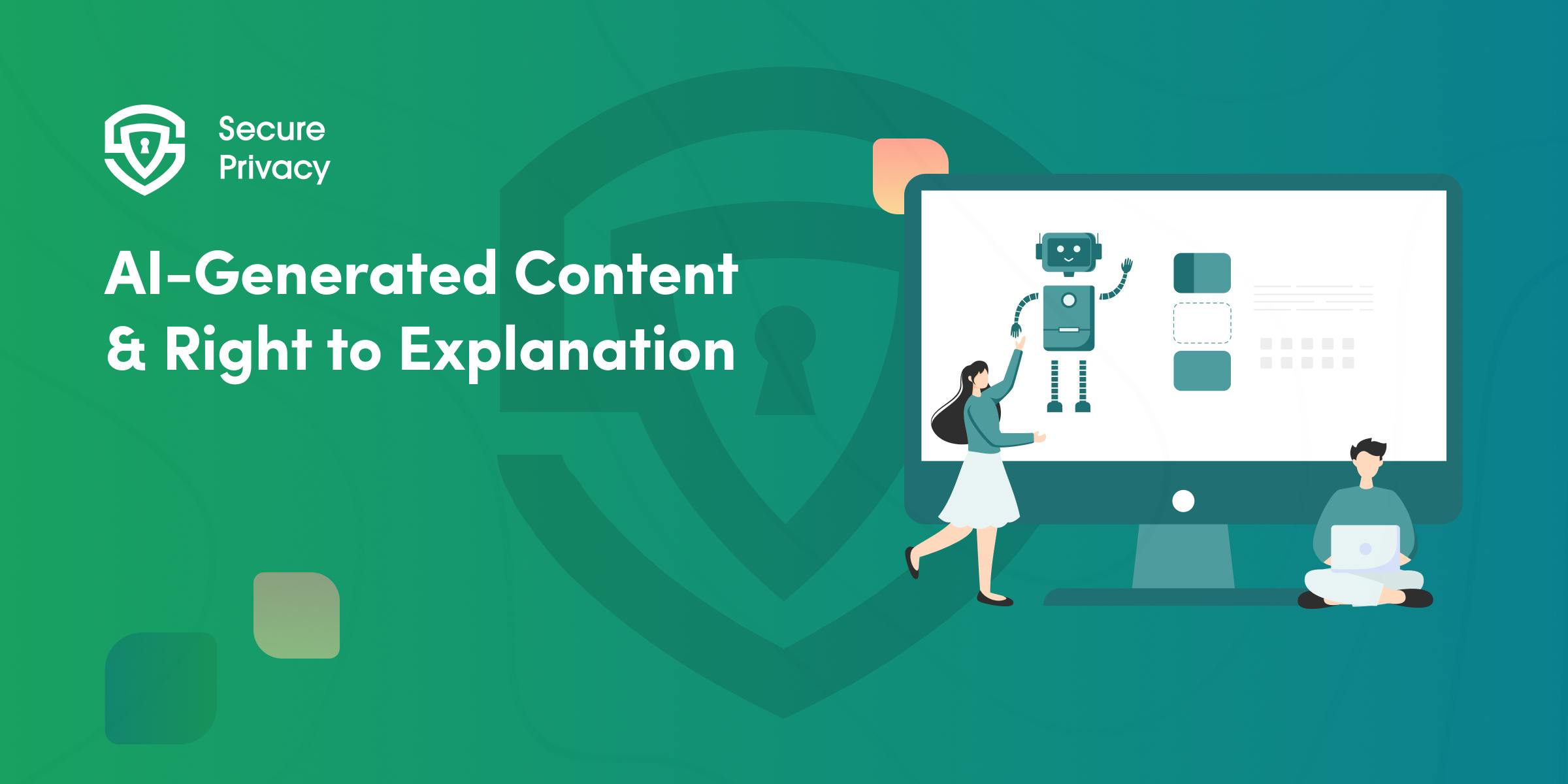
When AI Makes Decisions: Your Right to Know Why
You apply for a mortgage and get rejected. An algorithm flags your insurance claim as potentially fraudulent. A hiring system screens out your resume before human eyes ever see it. In each case, artificial intelligence made a decision affecting your life—but can you find out why?
- Legal & News
- Data Protection
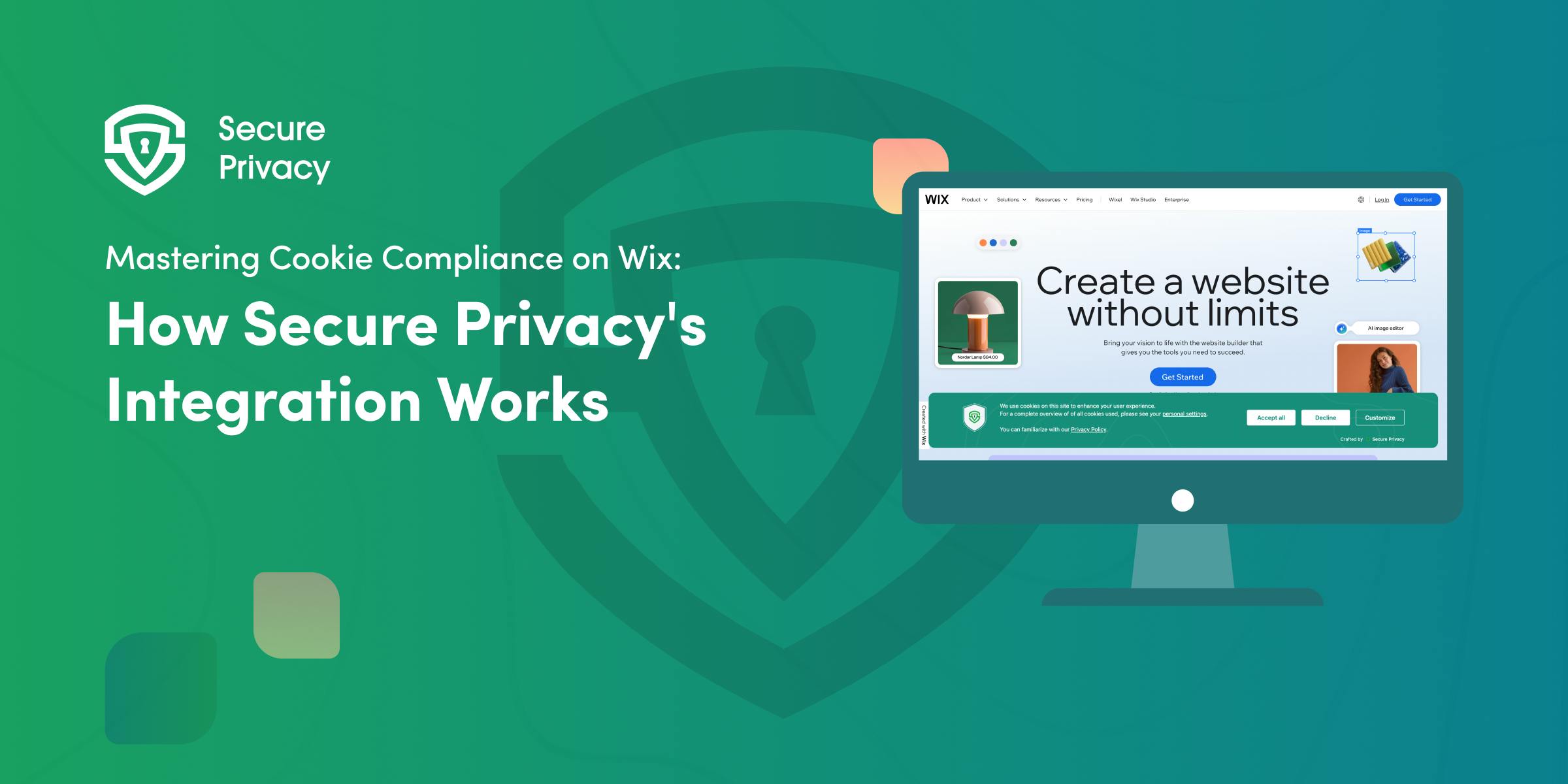
Mastering Cookie Compliance on Wix: How Secure Privacy's Integration Works
Your beautifully designed Wix website might be putting you at legal risk. As global privacy regulations tighten, that contact form, analytics dashboard, and social media widget all present potential compliance hazards without proper consent management.
- Legal & News
- Data Protection
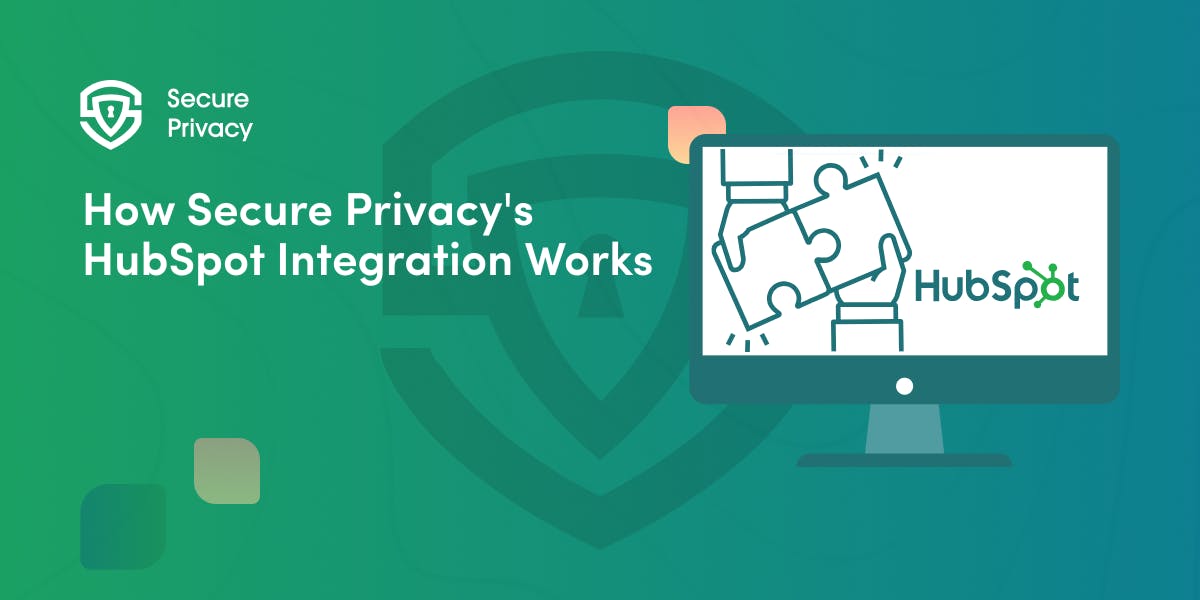
Cookie Compliance Made Simple: How Secure Privacy's HubSpot Integration Works
Your HubSpot website collects data with every visitor click, potentially creating compliance headaches under today's privacy laws. Without proper cookie consent management, you risk substantial fines—up to 4% of global revenue under GDPR alone. Secure Privacy's HubSpot integration offers a streamlined solution to this growing challenge.
- Legal & News
- Data Protection